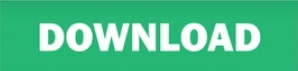
:max_bytes(150000):strip_icc()/GettyImages-490543806-67376302caa44996a7b9d590a7568f93.jpg)
Because LLMs generally require input to be an array that is not jagged, the shorter texts must be "padded" until they match the length of the longest one. Probabilistic tokenization also compresses the datasets, which is the reason for using the byte pair encoding algorithm as a tokenizer.
Benchmark testing clip art series#
Tokenizer: texts -> series of numerical "tokens" may be split into: An average word in another language encoded by such an English-optimized tokenizer is however split into suboptimal amount of tokens. Ī token vocabulary based on the frequencies extracted from mainly English corpora uses as few tokens as possible for an average English word. New words can always be interpreted as combinations of the tokens and the initial-set uni-grams. Token vocabulary consists of integers, spanning from zero up to the size of the token vocabulary. All occurrences of adjacent pairs of (previously merged) n-grams that most frequently occur together are then again merged into even lengthier n-gram repeatedly until a vocabulary of prescribed size is obtained (in case of GPT-3, the size is 50257). Successively the most frequent pair of adjacent characters is merged into a bi-gram and all instances of the pair are replaced by it. Using a modification of byte-pair encoding, in the first step, all unique characters (including blanks and punctuation marks) are treated as an initial set of n-grams (i.e. See also: List of datasets for machine-learning research § Internet Probabilistic tokenization Notable examples include OpenAI's GPT models (e.g., GPT-3.5 and GPT-4, used in ChatGPT), Google's PaLM (used in Bard), and Meta's LLaMa, as well as BLOOM, Ernie 3.0 Titan, and Anthropic's Claude 2. They are thought to acquire embodied knowledge about syntax, semantics and "ontology" inherent in human language corpora, but also inaccuracies and biases present in the corpora. Larger sized models, such as GPT-3, however, can be prompt-engineered to achieve similar results. Up to 2020, fine tuning was the only way a model could be adapted to be able to accomplish specific tasks. Īs language models, they work by taking an input text and repeatedly predicting the next token or word. Transformer architecture contributed to faster training. LLMs are artificial neural networks which can contain a billion to a trillion weights, and are (pre-)trained using self-supervised learning and semi-supervised learning. Its size is enabled by AI accelerators, which are able to process vast amounts of text data, mostly scraped from the Internet. If you're still having concerns, we recommend you check out /r/buildapc.A large language model ( LLM) is a language model characterized by its large size. If you're worried about CPU temperatures, please look at reviews for the laptop or CPU cooler you're using. Rule 6: CPU Cooling problems: Just like 95C is normal for Ryzen, 100C is normal for Intel CPUs in many workloads. This includes comments like "mUh gAeMiNg kInG" Please visit /r/AyyMD, or it's Intel counterpart - /r/Intelmao - for memes. Rule 5: AyyMD-style content & memes are not allowed. Commenting on a build pic saying they should have gone AMD/Nvidia is also inappropriate, don't be rude. AMD recommendations are allowed in other threads. i5-12600k vs i5-13400?) recommendations, do not reply with non-Intel recommendations. Rule #4: Give competitors' recommendations only where appropriate. No religion/politics unless it is directly related to Intel Corporation Rule 3: All posts must be related to Intel or Intel products. Rule 2: No Unoriginal Sources, Referral links or Paywalled Articles. If you can't say something respectfully, don't say it at all. This includes comments such as "retard", "shill", "moron" and so on. Uncivil language, slurs, and insults will result in a ban. Subreddit and discord for Intel related news and discussions.
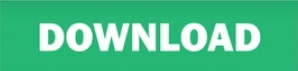